Multimodal AI Models
The emergence of multimodal models marks an exciting new frontier for AI. These models fuse text, images, audio, and other data to form a nuanced, multidimensional understanding of the world. Capable of identifying objects, people, actions, and emotions in visual media, these models do more than mere classification—they understand the relationships between objects and events. For instance, they can decipher the dynamics between characters in a movie scene or analyze traffic patterns in video feeds.
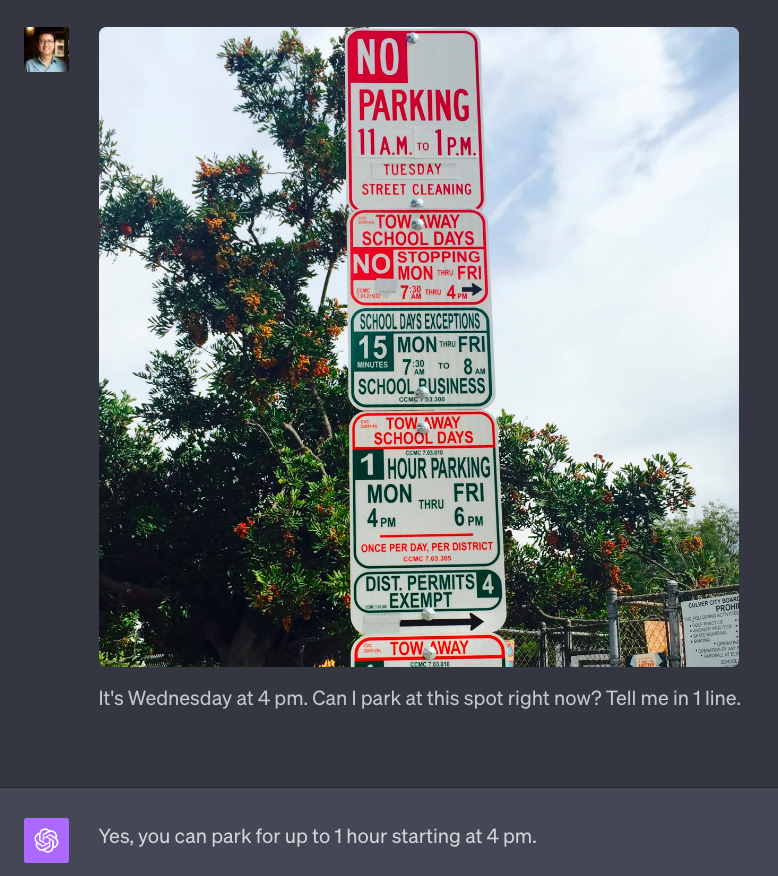
Other fields, such as robotics, that have struggled with computer vision could benefit greatly by using multimodal models as perception layer. For instance, Tesla is already using transformers to help navigate complex intersections.
In medicine, multimodal AI agents can help with diagnosis, by looking simultaneously at patient imaging data, test results and doctor notes.
However, they are some worrying possibilities too. Giving cameras the ability to interpret video in real-time paves the way for easy means of mass surveillance. Nation-states such as China that have an extensive, unified surveillance infrastructure can use this technology to monitor their entire population at all times.
Limits of Intelligence
In the near term, do not expect a sudden breakthrough in intelligence and reasoning beyond current GPT-4V levels. Auto-regressive models struggle with planning due to their design, which requires them to generate one token at a time sequentially. This limitation hinders their ability to plan beyond the next token. Moreover, since the selection of tokens is based on probabilistic methods from the training dataset, the models only operate within the scope of their training.
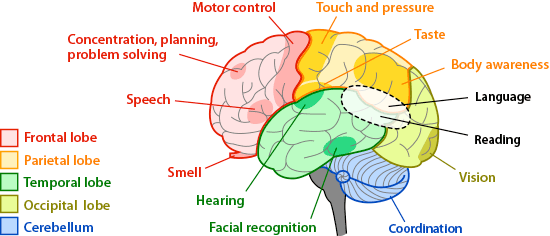
In a human brain, behavior is emergent from specialization and interaction between several distinct areas comprised of different kinds of neurons. Current AI models are generally homogeneous masses of neurons and at best, approximate small parts of the brain. Developing reason and planning capabilities most certainly will require a different cognitive architecture.
Promising behavior does emerge when multiple AI models collaborate, debate and refine their answer as a group (Source: MIT). Suggesting that fusing several models with different functions could be a path to higher level cognition.
Without a fundamental change in how AI models are structured, reasoning capabilities will remain limited.
Zero (Low) Cost Content Creation
100% AI Generated Movie
Generative AI is radically transforming the economics of content creation, reducing costs to nearly zero in many domains. This technology enables the rapid generation of diverse content, from written articles to visual graphics, without the traditional investments in time, expertise, and resources. For instance, AI can produce intricate designs, compose music, or write compelling narratives, tasks that previously required skilled professionals and considerable time.
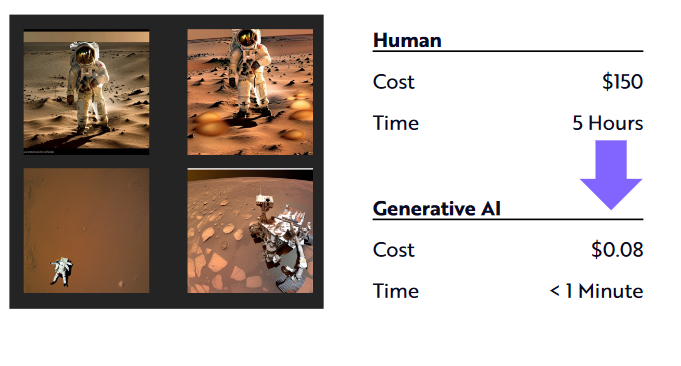
The drastic difference in production cost and time-frames will lead to rapid adoption of generative AI in graphics and video production. With a few commands, the AI could alter the location, seamlessly transporting the actors from a bustling cityscape to a serene mountainside, without the need for expensive and time-consuming location shoots. Lighting, often a painstaking task involving numerous crew members and equipment, could be adjusted with precision and variety, emulating the golden hour or the soft luminescence of twilight at the director's whim. Camera angles and movements, traditionally limited by physical constraints and the need for retakes, could be explored and perfected in real-time, offering an array of perspectives that would otherwise require extensive rigging and setup.
Cutting-edge AI models for images and videos are already yielding lifelike results. As this technology goes mainstream and attracts significant investment, we will see even better results.
News rooms are about to be disrupted with AI faster than you'd think.
— Greg Osuri ⟁ d/acc (@gregosuri) December 13, 2023
This broadcast is entirely generated by AI.
Hosts are NPCs anyway.
pic.twitter.com/FrrjFlzYvT
AI Everywhere
In 2024, we'll see generative AI start to be integrated into a wide range of everyday technologies. AI will take meeting notes, summarize documents, write blocks of software code and database queries, edit pictures, and intelligently link data models and APIs. AI-enabled apps will transform lives of people with disabilities, helping them see and communicate.
AI is slowly eating the traditional web. Compared to a web search, AI chatbots can provide answers more quickly and accurately. This shift is further propelled by the perceived decline of Google search quality and its increased focus on commercialization through sponsored results.
This presents a problem for content creators as well. The economics of the web has thus far been driven by advertising, but without human eye-balls scrolling through pages looking for answers, the business model no longer works.
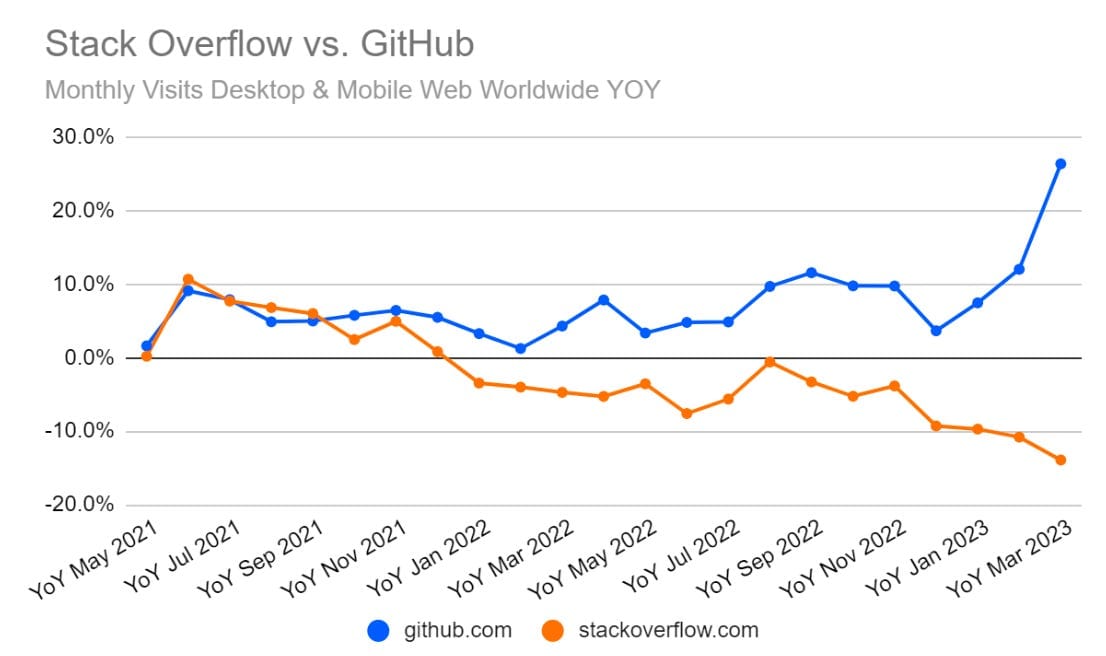
Recently, OpenAI inked an agreement with a publisher to summarize news articles from Politico, Business Insider and others. In the future, we'll see more content owners under pressure to ink agreements such as this one. At the same time, we will likely see the AI chat-bot role change from a passive responder to proactively "pushing" curated content to the user.
Hardware Supply Chain Risk
The AI hardware supply chain remains critically vulnerable. Major companies like Nvidia, Google, AMD, Tesla, and Apple don't manufacture their own chips and instead rely on TSMC to manufacture their designs. Because their production facilities are in Taiwan, the whole industry is susceptible to disruption from geopolitical tensions.

After years of cutting capacity, US is investing heavily in domestic production. In recent developments, Intel entered the foundry services business and is on track to open a new Arizona facility in 2024. Samsung aims to beef up AI chip foundry sales to about 50% of its total foundry sales in five years.
Company | Country | Q2 revenue, in billions | Market share |
---|---|---|---|
TSMC | Taiwan | $15.66 | 56.4% |
Samsung | S. Korea | $3.23 | 11.7% |
GlobalFoundries | U.S. | $1.85 | 6.7% |
Expect continued significant investment in semi-conductor fabrication capacity as countries see AI critical to national security. Stressed water and energy supplies will likely dictate where these plants are built.
Competitive Landscape
Although OpenAI will likely maintain its position as the market leader, competitors are not far behind. For instance, Anthropic's Claude 2.1 is capable of processing inputs up to 500 pages or 200,000 tokens, surpassing GPT-4's 128,000-token limit.
Open-source models are becoming more compact and efficient, with several now operable on consumer-grade devices. Running models locally offers enhanced privacy, reduced latency, and lower costs. Apple will likely take this approach as they prepare to enter the market imminently (Apple: LLM in a flash).
In what is becoming a popular trend, users are starting to fine-tune open-source models for their own domain specific applications. When fined-tuned in narrow use cases, smaller LLMs can match large proprietary models. Mistral 7B Fine-Tune Optimized can even surpasses GPT-4 in certain benchmarks.

There's notable progress in training methodologies, with smaller, higher-quality datasets leading to more efficient and reliable models. Microsoft's Phi-2, with just 2.7 billion parameters, outperforms the much larger Llama-2 70B model in various tasks.